Unlocking the Power of Multimodal Learning for Emotion Recognition in Conversation
Abstract
Emotion recognition in conversation aims to identify the emotions underlying each utterance, and it has great potential in various domains. Human perception of emotions relies on multiple modalities, such as language, vocal tonality, and facial expressions. While many studies have incorporated multimodal information to enhance emotion recognition, the performance of multimodal models often plateaus when additional modalities are added. We demonstrate through experiments that the main reason for this plateau is an imbalanced assignment of gradients across modalities. To address this issue, we propose fine-grained adaptive gradient modulation, a plug-in approach to rebalance the gradients of modalities. Experimental results show that our method improves the performance of all baseline models and outperforms existing plug-in methods.
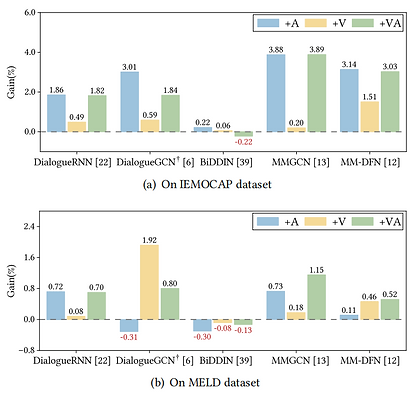
Medol
To ameliorate the challenge of imbalanced gradient assignment in the ERC task, we introduce an innovative Fine-grained Adaptive Gradient Modulation (FAGM) mechanism. This strategy aims to rebalance gradients across modalities, placing emphasis on enhancing the optimization of suppressed modalities by modulating the gradients according to their respective dominance proportion. However, discerning the gradients of distinct modalities within multimodal models is intricate due to the shared parameters across modalities. To navigate this complexity, we pioneered the Modal Parameters Decoupling (MDP) technique that identifies parameters endemic to specific modalities. This method involves assessing the sensitivity of each neuron across modalities, subsequently classifying parameters based on their sensitivity. This refined approach facilitates a rebalance of modality training at the parameter level, culminating in enhanced optimization and bolstered the performance of multimodal models in the ERC task.

Experiment
To qualitatively validate the effectiveness of our proposed method, we displayed the decoupling losses of textual and audio modalities before and after modulation on partial models in Figure 6. According to the analysis in Section 4, the textual modality is the dominant modality in training and suppresses the optimization of audio modality. It can be observed that, after modulation, the optimization of audio modality is more sufficient, while the optimization of textual modality is suppressed slightly. Furthermore, in Figure 7, we displayed the dominance proportion computed by Eqn. (3), which can reflect the degree of each modality dominates model training. After modulation, the proportion of textual modality slightly decreases while that of visual modality slightly increases. Therefore, This shows that our method effectively balances the optimization of different modalities, ensuring that slower-optimizing modalities are fully optimized.
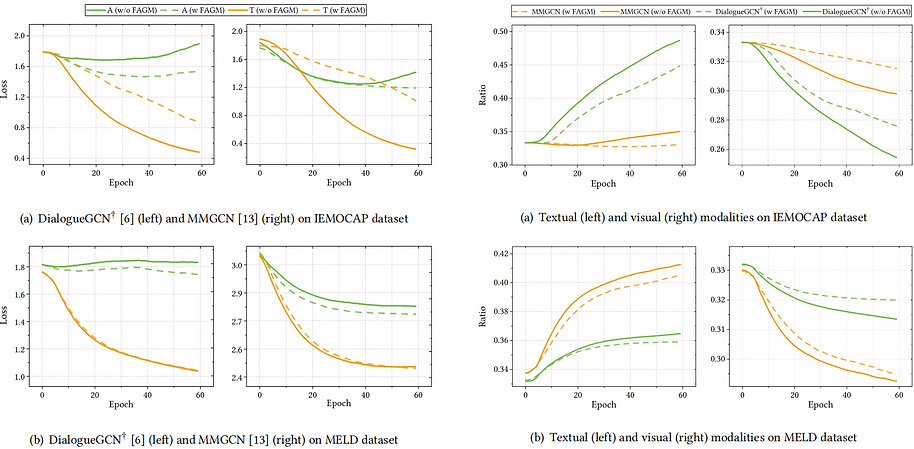